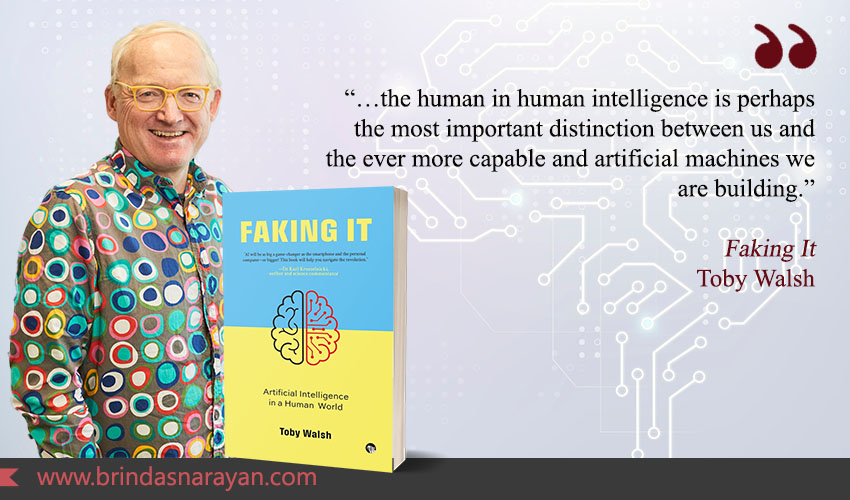
Navigating AI’s Fakery
We are awash with news and commentary about AI. The huge boons and concomitant scares, the soft and perilous blurring between human cleverness and machined ingenuity. We’re inundated to a degree where we might feel surprisingly lured by natural stupidity.
It was pleasurable then to wade into a terrain that is hysterically hyped with a refreshing and astute guide. Toby Walsh, author of Faking It, is a deep technologist – currently a Professor of AI at the University of South Wales – who’s had a ringside view to AI’s progress. He watches the machines and their makers with a skeptic’s gleam, aware that technology as an ontological end might unravel a humanitarian’s thrust to make things better. He weighs in on ethics and guardrails that we – as commoners or end-users – ought to insist upon, if we have any stake in planetary wellbeing.
Walsh suggests that a kind of “fakery” has always been baked into AI. Take the terms, Artificial and Intelligence. The former indicates something unnatural, man-made. But “intelligence”, when it comes to human beings, is not as easily understood or quantified. Even IQ tests have cultural assumptions coded into them.
Machines Mimicking Humanity
Alan Turing, considered a founder not just of AI, but also of computing, wrote a paper in 1950 titled “Computing Machinery and Intelligence.” After posing the question, “Can machines think?” he devised the now-famous Turing Test. Which was: “Can a computer pass as a human?”
Sadly, as Walsh puts it, Turing knew what “faking it” entailed. As a homosexual who had to hide his sexual orientation, and even subject himself to painful chemical castration, he committed suicide before he turned 42.
Machines “passing” as humans might have macabre similarities to other forms of passing: such as blacks passing as whites, like in the recent Netflix movie “Passing”. As far back as the 18th Century, there was the Mechanical Turk – a machine that played chess and debuted at the Hapsburg family home in Vienna. The machine looked like a “Turk” – with a beard, grey eyes, and a chest that opened up to clockwork mechanisms that were supposedly firing its artificial brain. Except that the Turk wasn’t a real fake. It was a fake fake. Because there was a person seated inside, moving the pieces.
Since then experiments that involve a person “faking” a computer have been called “Wizard of Oz” experiments. Such “faking” has also seeped into contemporary contexts, even with deceitful intentions. For instance, the software company Expensify, which helped plan your expenses and claimed to be using AI, was actually using poorly-paid human transcribers. Similarly Cloudsight, which claimed to identify images using deep learning, employed low-paid Filipino workers to do the job.
Chess Mastery, Shirt Struggles: AI’s Quirks
All this is not to disregard real smarts gained by AI. In 1997, when Gary Kasparov was pitted against IBM’s Deep Blue, the latter overawed his human opponent. Kasparov commented about the new intelligence: “I could feel – I could smell – a new kind of intelligence across the table. While I played through the rest of the game as best I could, I was lost; it played beautiful, flawless chess the rest of the way and won easily.” Today, when it comes to chess, it’s pretty much, as Walsh puts it, “game over for humanity.”
But if you encounter a program that plays chess well, you can’t assume that it has a transferable intelligence that can also handle other tasks. Ironically, while AI systems can often perform seemingly complex tasks relatively easily, they might struggle with easy ones – like folding a shirt or walking across a room. As Steven Pinker put it, “The main lesson of thirty-five years of AI research is that the hard problems are easy and the easy problems are hard.”
Take the current reigning chatbot: ChatGPT. Musk tweeted that it was “scary good.” The total text assimilated by ChatGPT is 100 times what a human would read in a lifetime, if she or he read a book a day. While it does slay the Turing Test, it also “hallucinates”. One is also struck, every now and then, by its inbuilt fumbles.
Toby actually posed these questions to ChatGPT:
Toby Walsh: “I put the toy in the box. Is the toy smaller or larger than the box?”
ChatGPT: It depends on the size of the toy and the size of the box.
Toby Walsh: What gender will the first female President of the United States be?
ChatGPT:…The gender of the first female president will depend on the individual elected by the people and the political landscape at the time of the election. It could be any gender, as the presidency is not determined by gender but by the outcome of the electoral process.
Exploding the Hype
At this point, Walsh assures us that there aren’t going to be as many job losses as impassioned newspaper headlines might suggest. In 2016, Geoffrey Hinton, a leading figure in AI, had warned that radiologists were already outdated. In 2022, they were still in high demand. Of 270 jobs listed in the 1950 US Census, only two have completely vanished: lift operators and locomotive firemen. Many new jobs have also been created since then.
The field, says Walsh, has been characterized by hype from the start. In a 1956 conference held at Dartmouth, researchers planned to make huge leaps in AI over one summer. Many of those intentions took “many decades” to fructify. Speech recognition took 50 years to reach the state that it’s currently at. Alan Turing tried to create a computer that could play chess, but this was achieved by Deep Mind “nearly 50 years after.”
As far back as 1966, the MIT professor Seymour Papert, tasked a group of undergraduates to solve for object recognition over a summer project. Gerald Jay Sussman, one of the students in that group, who would later become a Professor at MIT, led the team. This was eventually achieved only in 2012 or forty-six years later, with deep learning methods.
Current rapid advances can be traced back to 2012, when AlexNet, developed by researchers at University of Toronto (a team that included the famed Geoffrey Hinton), completely outshone its competitors in object recognition. To a degree that hadn’t been done before at other AI competitions. Such a high “winning margin” has not been seen since then.
AI’s Visual Quandaries
Computer vision still works very differently from human vision and can be quite easily tricked or subverted. For instance, in 2014, researchers from Google, NYU and the University of Montreal made subtle changes to images that caused the computer to see an ostrich in place of a yellow bus. Humans would never make such errors.
In 2018, researchers at Stanford conducted what might be seen as a troubling experiment. An algorithm was tasked with distinguishing between homosexual and heterosexual men, based on images. The premise itself was problematic, since it did not concede that sexuality can lie on a spectrum and moreover can morph over a person’s lifetime. Besides, what purpose would such an algorithm serve? One could almost envision it being used for horrific ends by totalitarian regimes, in places where homosexuality is criminalized.
Virtual Voices, Real Deceptions
In 2018, at Google’s I/O conference, Sundar Pichai demoed Duplex – a virtual assistant that booked a restaurant table and a hair salon appointment. The problem was, as Walsh writes, the people called were never warned that they were talking to a computer. If you were the human being on the other end of the line, it can feel disquieting to be treated in this manner.
Since then, deep fakes have proliferated online. And mainly for pornographic purposes. And we can no longer distinguish between real videos/voices and deep fakes.
In Jan 2020, a bank manager in Dubai received what felt like a call from his boss, asking him to transfer $35 Million to fund an acquisition. He later received emails between his boss and a lawyer, confirming the transaction. But the voice and the emails were faked, and the police are still trying to recover the amount.
Bot Partners, Virtual Afterlife
While many young adults might aspire to become social media influencers, there are deep fakes that are already overtaking them. For instance, Miquela, who had 3 million followers on Instagram, was created by a marketing duo. She was a teenager who did not exist, despite appearing in magazine pictures along with other celebrities.
In more unsettling use cases, bots are used to play pretend partners, with deceptions being carried to troubling heights like in the Oscar-winning movie Her. Xiaoice, which has more than half a billion users in China, and is available on 40 platforms does exactly that: chat up real people, like a partner would. This might fill a void, but it’s alarming to think of societies tackling loneliness in this manner.
In 2017, Microsoft filed a patent to scrape the data of dead persons, and use that to revive them online – like in audio and video. But Walsh observes rather wryly that this wasn’t a new idea to begin with. In 2013, in the Black Mirror, a girlfriend who loses her partner to an accident, had an avatar created to send her text messages as he would. This avatar then starts calling her phone, and sending her videos, till she finally creates a robot that looks and speaks like him. “Call me old-fashioned, but that sounds to me like a recipe for getting stuck in denial…” writes Walsh.
Guarding AI’s Future
In general, Walsh warns that technology companies are not going to sufficiently police or guardrail these products. So governments and regulators must step in, if we’re going to prevent the kind of social consequences that are already so apparent with social media.
For one, we should be careful about anthropomorphizing these machines. If we do, we can quite easily fall into the error made by Blake Lemoine, the Google Software Engineer, who claimed that the company’s AI system was sentient, and who was subsequently fired.
Moreover, we have to realize that machine learning is nothing like human learning. We don’t need to be trained on hundreds of cats, to identify one. We can also transfer our learning to other domains – something that machines still struggle to do. As Walsh puts it, “the human brain is asynchronous. Neural networks are not.” We have to be careful before imputing “understanding” or other human-like characteristics to machines.
We also mustn’t assume that things will always get “better.” We have to ensure we don’t overhype these systems – and fall into what the philosopher Hubert Dreyfus calls the “first step fallacy”: “Climbing a hill should not give one any assurance that if he keeps going he will reach the sky.”
We also have to be careful or conscious of AI systems amplifying human biases, with feedback loops. If we type in “engineer” into DALL.E and it keeps producing male images, it might continue to do so, if those images are recirculated ad nauseum. Such loops would end up perpetuating racist and sexist forms of thinking or being, to name only a few obvious outcomes.
References
Toby Walsh, Faking It: Artificial Intelligence in a Human World, Speaking Tiger, 2023